Using high-resolution imagery and deep learning to classify land-use following deforestation: a case study in Ethiopia.
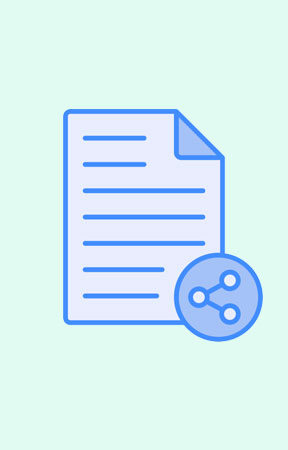
This study evaluates the potential of satellite imagery for classifying land-use after deforestation in Ethiopia using an attention-enhanced U-Net deep learning model. Single-date high-resolution images (Planet-NICFI) or multi-date moderate-resolution imagery (Sentinel-2, Landsat-8) were most effective, while medium-resolution single-date data underperformed. Adding attention mechanisms improved accuracy, particularly for small-scale land-uses. The findings highlight the importance of sensor selection and data integration for land-use classification, supporting more effective deforestation monitoring and data-driven policymaking in Ethiopia.
Other:
https://robertnag82.users.earthengine.app/view/deforestationdriverethiopia;
https://zenodo.org/records/11061499;
https://github.com/masolele/Attention-U-Net-4-landuse-mapping-Ethiopia